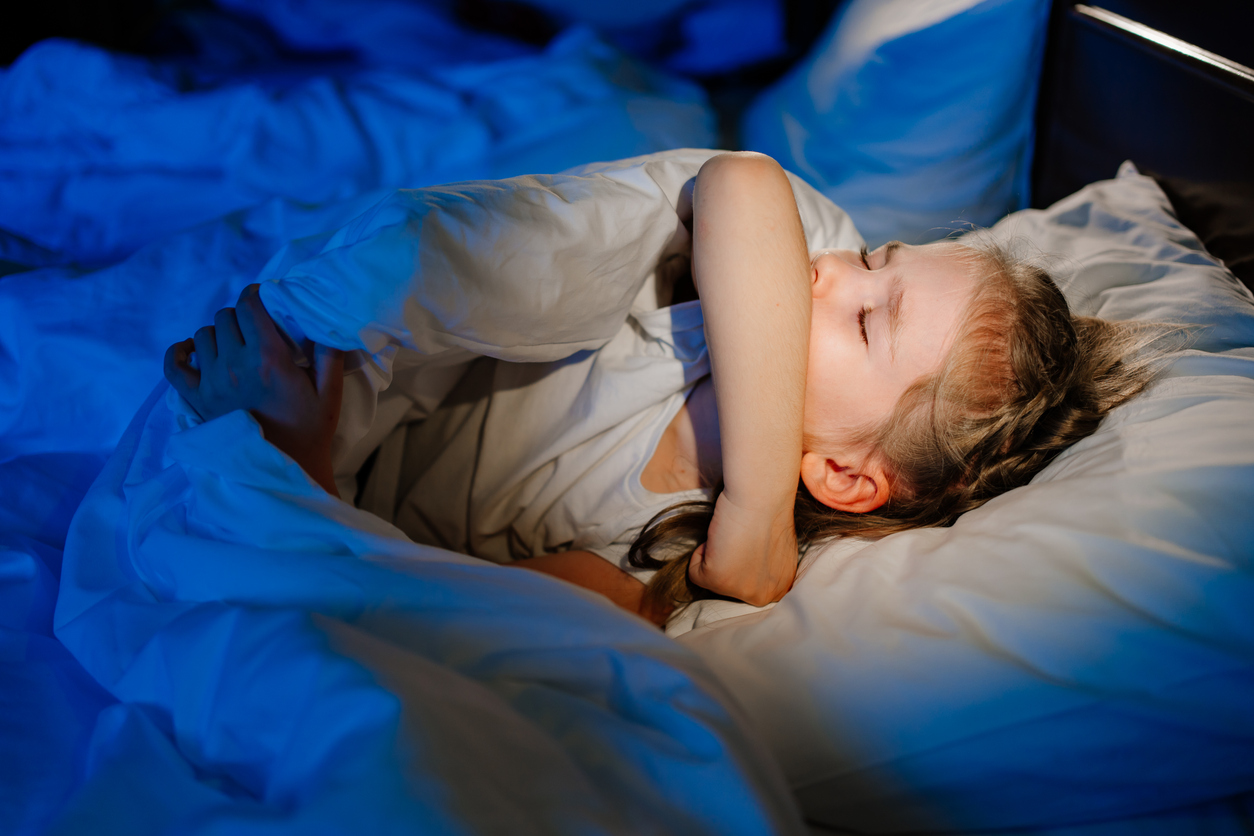
This blog provides a brief summary of a recent study "Children with severe asthma have substantial structural airway changes on computed tomography" by Wytse B. van den Bosch et al. (ERS Journal, Jan'2024), highlighting key insights and potential impact on treatment of the disease.
Around 10% of children with asthma are classified as severe. This means they continue to suffer from severe asthma symptoms despite adequate anti-asthma therapy. The reason why is mostly not clear. In many of these cases a lung CT is made to evaluate whether there is damage to the lung structure and to exclude alternative diagnosis. The evaluation performed by expert radiologists and pediatric pulmonologists is traditionally done by eyeballing. Unfortunately, this visual interpretation of the images is insensitive to thoroughly assess the condition of the hundreds of bronchi that are captured on a CT scan. Typically, this evaluation is based on around 20-30 mostly larger bronchi which can be assessed visually. The more experienced the specialist, the more accurate the assessment.
Thanks to the development of fully automatic image analysis systems enabled by artificial intelligence, it has become now possible to measure the dimensions of almost all airways and blood vessels on a CT scan, with great precision and accuracy.
Seeing the 'unseen', measuring the 'unmeasurable'
In the study by Bosch et al Thirona’s LungQ software was used successfully to assess CTs of 131 children with severe asthma, treated at the Erasmus Medical Center Rotterdam. Over 20’000 bronchus-blood vessel pairs were measured, including a large number of small bronchi which otherwise could not be visually assessed by a human. While LungQ conducted the measurements of bronchus-artery ratios on each CT in minutes, doing this by hand would require up to one week per CT by a well-trained technician.
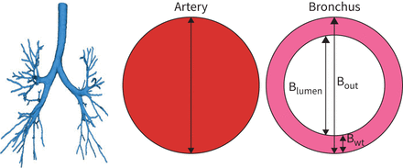
LungQ™ Bronchus-Artery Analysis
LungQ-BA software segments the bronchial tree, identifies the generation for each bronchus–artery (BA) pair and measures the following BA dimensions: outer bronchial wall diameter (Bout), adjacent artery diameter (A) and bronchial wall thickness (Bwt).
Unexpectedly, it was shown that almost all these children have substantial abnormalities of airways, with bronchial dilatation (defined as bronchiectasis, currently thought to be irreversible) detected in one third of the patients. These findings are of great value for enhancing our understanding of severe asthma and for learning more on the development of bronchiectasis disease as diagnosed in adults. The ability of artificial intelligence to assess changes in bronchial dilatation and bronchial wall thickening with high sensitivity, can potentially help uncover new insights on the development of permanent airway damage.
Furthermore, it opens the possibility to use lung CT images to evaluate the effectiveness of novel therapies targeted at improving the condition of the airways and even potentially preventing the development of irreversible impairment.
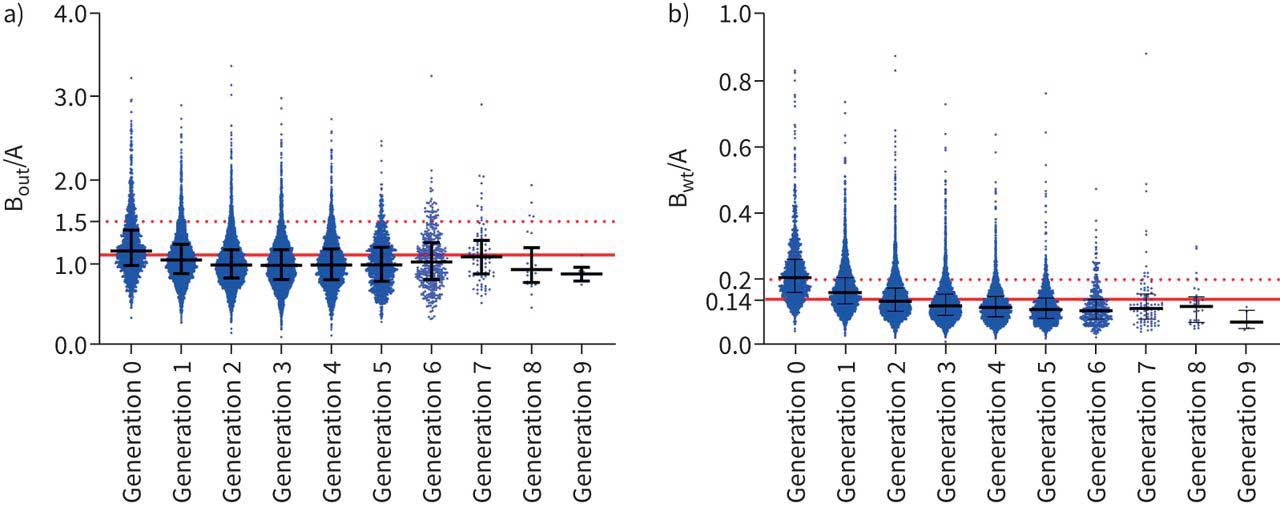
LungQ™ Bronchus-Artery Analysis
Bronchus–Artery ratios measured by LungQ-BA software. Blue dots represent individual measurements of the Bout/A, and Bwt/A ratio. The two figures show the relation between the different measurements, starting at the segmental level (generation 0) up to the 9th segmental generation, deep down in the lungs. Dots above the solid red line are dilated (Bout/A) or thickened (Bwt/A).
Finally, the study underlines the importance of routinely including a lung CT with automatic analysis of the images to ensure objective and sensitive assessment of airway damage in patients with severe asthma and to personalize treatment and monitoring.
The ability of artificial intelligence to assess changes in bronchial dilatation and bronchial wall thickening with high sensitivity, can potentially help uncover new insights on the development of permanent airway damage
The ability of artificial intelligence to assess changes in bronchial dilatation and bronchial wall thickening with high sensitivity, can potentially help uncover new insights on the development of permanent airway damage
Quantitative evidence enabled by artificial intelligence
Severe asthma in children is relatively rare. Although after addressing modifiable factors such as treatment adherence, comorbid conditions, and harmful exposures, children whose asthma remains poorly controlled despite adequate medications display a diverse range of severe asthma phenotypes. In recent years, significant progress has been made in understanding the underlying mechanisms of severe therapy-resistant asthma in children. Nevertheless, asthma attacks and hospitalizations remain frequent. Furthermore, severe asthma can be a misdiagnosis as other conditions can result in similar symptoms. Thorough evaluation and characterization of each child’s disease conditions, based on comprehensive quantitative evidence enabled now by artificial intelligence, will be essential to ensure the most effective and suitable treatment approach, enhancing the quality of life of children with severe asthma.
Feel free to contact Thirona’s expert team to learn more on our capabilities in bronchi and mucus analysis.