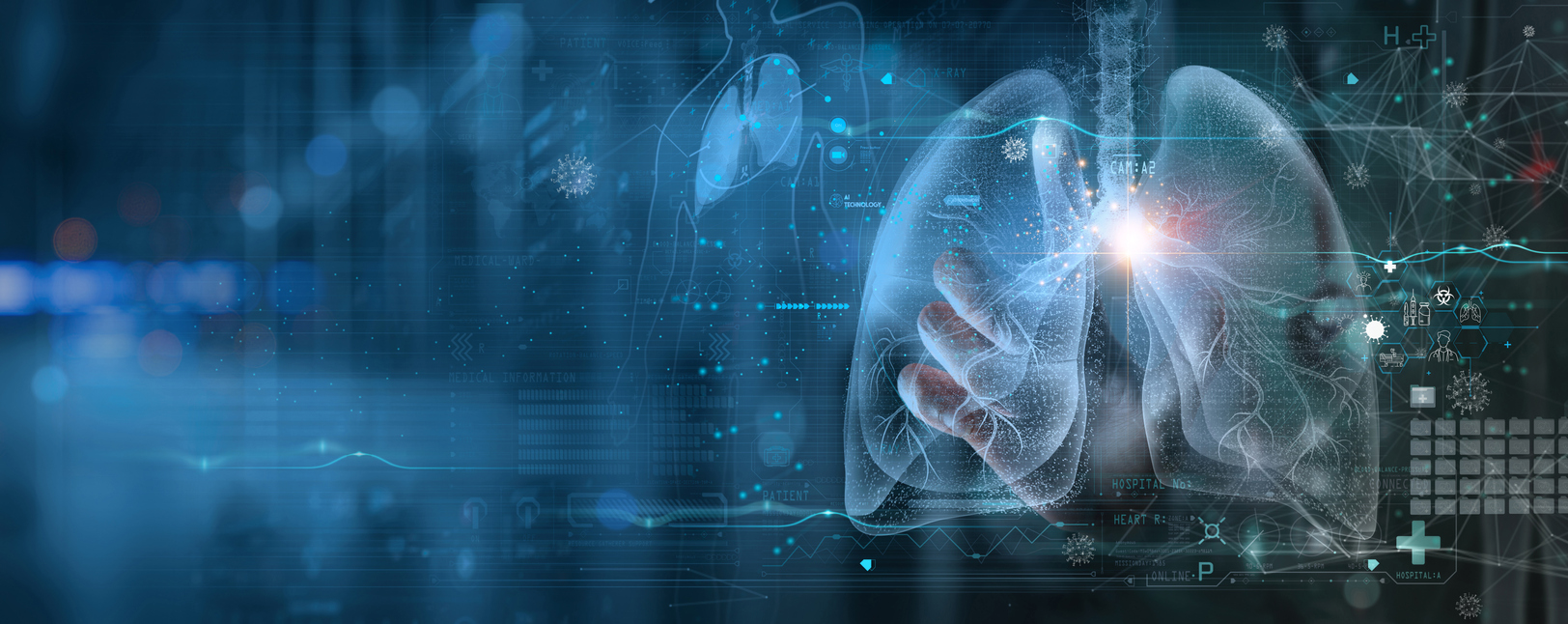
Jean-Paul Charbonnier, CIO of Thirona explains 4 critical steps to effectively applying AI-enabled lung image analysis in pulmonary clinical trials.
Why do so many trials struggle with getting it right?
Quantitative evaluation of medical images with artificial intelligence (AI) plays an increasingly important role in clinical trials for drug and treatment development. Especially for pulmonary diseases, chest CT image analysis can help improve trial outcomes and provide robust imaging endpoints, as well as uncover new insights into disease progression and mechanism of action. Enabling objective and precise quantification of parenchymal abnormalities, airways, or pulmonary blood vessels, AI revolutionizes the way we can assess otherwise difficult to evaluate structural changes and pathologies such as bronchial wall thickening, mucus impaction, pulmonary arterial enlargement, pulmonary fibrosis, or hypoperfusion.
Clinical trials are time consuming and expensive to complete. Using imaging to assess a trial’s primary, secondary or even exploratory endpoints, not only helps to provide more accurate and precise outcomes but can also provide interesting new insights for future trials, even in small patient populations. But why do so many trials struggle with getting it right? Why do some imaging trials still fail while others deliver robust evidence enabling breakthrough innovations?
Through eight years of experience in working with Pharmaceutical and MedTech companies, having analyzed 15 million medical images, we have been able to recognize typical challenges to apply image analysis effectively. Failing to deliver statistically strong evidence is often not based on the performance of an algorithm. The cause is frequently rooted in the choice of a set of biomarkers in the design phase of a trial, particularly in the context of specific diseases and treatment effects. Furthermore, insufficient image data quality and data inconsistencies often make it challenging to deliver robust and reproducible results, especially in a longitudinal setting where patients are followed over time, or in multi-center studies where data is analyzed from a range of different sources.
Based on our experience, we have observed the following three top challenges:
- Managing and handling patient and scanner variability of incoming imaging data;
- Selecting the best-fit-for-purpose measurements, optimally matching the study’s requirements;
- Delivering consistent and reproducible output quality for large-scale longitudinal trials.
In this article, I will share key learnings on how to use quantitative CT image analysis to its best potential and boost the reliability of clinical trials. We see the following four steps as critical to any trial that considers using imaging:
- Precise understanding of the treatment mechanism, treatment outcomes and patient population;
- Understanding the advantages and disadvantages of each quantitative biomarker and selecting the best fit-for-purpose imaging biomarkers;
- Handling image quality and data variation for reproducibility of the results;
- Tailoring the study to specific patient populations and applying effective inclusion criteria.
1. Understanding the trial’s full picture
Quantitative image analysis can play several roles within a clinical trial. Quantitative analysis of baseline scans, for example, can be used to identify certain patient inclusion and exclusion criteria, characterize a patient population, or aid in the selection of specific treatment targets. During the follow-up phase of a trial, quantitative markers can play a powerful role in precisely measuring structural changes or disease progression over time linked to a particular drug or intervention.
Ensuring high reliability of trial outcomes starts with a thorough understanding of the trial objectives and treatment mechanism of an intervention or a drug. By understanding these details, the best-fit biomarkers can be selected to deliver evidence for treatment effectiveness.
Different diseases, disease interventions, and trial goals require a different set of biomarkers and AI algorithms. To make it even more complicated, markers can be extracted on a global level (lungs), on a more regional level (lobes, segments, subsegments), or even down to the voxel level of the image. Defining the optimal approach for the quantitative assessment therefore goes beyond just selecting a good biomarker.
At Thirona, for example, we work closely with Pharmaceutical companies, MedTech companies, or CROs, to get a deep understanding of the treatment and the treatment effects that trial sponsors are trying to measure. This understanding, combined with our expertise on what information can be extracted from CT images, forms the basis for selecting the best image analysis method for the trial.
2. What exactly do we want to measure, and what can be measured?
There is a whole collection of CT derived outcomes that can be extracted in relation to pulmonary function and disease. We typically divide pulmonary analyses into three main categories related to: the parenchyma, the airways/ventilation, and the pulmonary vasculature/perfusion. Depending on a trial’s goal, we dive into possible measurements within those three areas to define the most appropriate set of biomarkers. When it comes to defining customized measurements for novel treatment solutions, we source from our extended AI platform with a wide range of validated and innovative biomarkers, such as quantification of the airway wall thickness to measure efficacy of mucus-reducing drugs; volume quantification of the pulmonary arteries to assess the severity of pulmonary hypertension; or precise identification of a pulmonary sub-segment to inform bronchoscopic interventions and pulmonary segmentectomy. A good example of a recent innovation is our PXT biomarker [1], which could replace a SPECT-CT scan for selection of viable target regions in bronchoscopic interventions in several COPD treatments. This enables an AI-supported analysis of just a single chest CT image, directly at the hospital, without the need for an additional scan, saving both radiation exposure to the patient and reduces time and cost associated with the procedure.
In some cases, a clinical trial has already clearly defined biomarker requirements. Still, adding certain exploratory outcomes can help discover important new insights into the effectiveness of the intervention, and to design subsequent trials. An interesting example is the COPDGene study (the largest COPD study in the US involving more than 10,000 patients), for which Thirona has been running the quantitative image analysis for the entire main study. While COPD studies are generally focused on the development of emphysema and airways remodeling, we have additionally explored pulmonary vascular markers to investigate the involvement of the pulmonary arteries and veins in the development of COPD. The ability to quantify pulmonary blood vessels, while distinguishing between arteries and veins, may lead to new insights into the complex pathophysiology of COPD and potential new treatment areas.
A typical pitfall we have encountered is that trial designs often default to “what was done in previous studies,” without considering new abilities in the field of quantitative imaging. This is why in some longitudinal cohort studies, visual assessment methods or out-date quantitative analysis are still being used to compare results from one study to another. While visual reads by clinicians can provide qualitative assessment that offers some insights into characteristics associated with clinical response, it is less suitable for identifying small changes or providing the extent of change, when compared to quantitative image analyses with AI. In this respect, retrospective reanalysis of CT scans from earlier trials still can provide additional information, speeding up future innovations.
A recent example of such retrospective analysis is a study performed in Cystic Fibrosis (CF). In CF, CT scans can monitor clinically relevant structural airway changes over time with great precision, which provides invaluable information for patient care. As the recent SHIP-CT study [2] showed, trials for CF drugs under development may benefit from using CT as part of the primary or secondary endpoint. In this study, quantitative CT analysis provided information of the exact changes that were occurring in the airways, while visual reads of the original study were not sensitive enough to pick up this signal.
3. Reproducible Image Quantification – Handling Data Variation
CT scanners and acquisition protocols generally vary from site to site. These variations may lead to inconsistent imaging data, which in turn can influence the interpretability of the CT scans. When not handled properly, it could strongly impact the quantitative assessment of a scan. Standardization of CT protocols is particularly important in multi-center trials, due to the inherent variability of using different scanners, and in longitudinal trials to ensure that the data is translatable across patients and time points.
There are several ways to substantially mitigate CT data variation at the source:
- Securing a strict standardized imaging protocol for the clinical sites to follow;
- Performing rigorous quality checks on the data and flagging deviations of the protocol in an early stage;
- Ensuring a quick follow-up with the clinical sites for correcting the deviation in early stages of the trial.
Nevertheless, obtaining consistent CT image quality during the length of a trial is not always possible. Despite strictly defined protocols, it is not uncommon to have scanner drifts or scanner upgrades take place during a long longitudinal trial or CT variation as a result of human error. Also, analysis of retrospective data, such as already acquired data in a rescue trial, generally lacks well standardized imaging protocols.
Therefore, in addition to image acquisition standardization, there are several post-acquisition measures that can be put in place during the quantitative analysis phase:
- Using image normalization techniques to ensure that the CT data is normalized before the quantitative analysis;
- Ensuring that the quantitative algorithms used in a trial are designed to handle data variation;
- Selecting biomarkers that are less vulnerable to differences in acquisition protocols.
Regularly supporting ‘rescue trials’ at Thirona, we often witness how inaccurate standardization of imaging protocols compromises the ability of a trial to achieve its objectives – eventually resulting in failure. To help solve this problem we have developed several normalization algorithms to optimize image quality for image variations caused by scanner difference, dose protocols, or image reconstruction settings. The “Normalized emphysema scores on low dose CT: Validation as an imaging biomarker for mortality” [3] study is a good example of how image normalization in a multi-center study was of crucial importance to find a significant mortality signal.
When the clinical trial is well-controlled with high data consistency, a successful trial can eventually lead to implementation in clinical care. Unlike clinical trials, data from clinical care can have a higher variability as standardization guidelines are not globally implemented. Therefore, decisions on the imaging protocol and image analysis for a trial may impact later translation to a clinical environment, for example when using scanners that are not widely available or applying biomarkers that are not well suited for a wide clinical population. Robust normalization techniques and AI algorithms trained on a wide patient population, taking diseases and image variation into account, are thus crucial to make the solution work reliably in a clinical environment. Thirona’s expertise in handling data variation enables us to support translation of pivotal algorithms that are successfully used in clinical trials, into regulatory certified applications for clinical use. Several solutions are already clinically used in more than 30 countries for routine clinical interventions like, for example, endobronchial Zephyr® Valve [4], [5], FreeFlow® Coil System, or for PulmoVR [6]that has recently entered a multi-center trial phase.
4. Patient and target Selection – For Some Trials the Make-or-Break Factor
As we have learned from experience, whether it is a pharmaceutical company testing a new drug, or a MedTech company evaluating performance of a new medical device, selecting the right patients and treatment targets can simply make or break the success of a trial. Increasingly, part of the patient inclusion can be done with quantitative scores from CT scans.
So, how do you select the right patients or the best treatment targets to ensure a successful trial? Again, it all starts with a deep understanding of the patient population, related disease pathologies, and treatment mechanism of a drug or device. For example, when treating a chronic bronchitis patient with mucus reducing therapies, it is important that these patients have indeed excessive mucus production in the airways. This can be well visualized and quantified with CT, which can even be used to localize the best treatment locations in case of a targeted intervention.
There is growing evidence from scientific literature that highlights the importance of patient selection. Lung diseases are generally very heterogeneous, meaning that a successful treatment may eventually only be found in a sub-group of the target population. For example, the results of the study, Predictors of Response to Endobronchial Coil Therapy in Patients with Advanced Emphysema [7] showed that quantitative CT analysis is critical for patient selection and treatment planning for endobronchial coil therapy. Researchers concluded that the addition of quantitative CT, is key in excluding patients with relevant airway disease, who are less likely to benefit from coil treatment.
Another interesting example of the need for treatment target selection is the study “Reversal of collateral ventilation using endoscopic polymer foam in COPD patients undergoing endoscopic lung volume reduction with endobronchial valves: A controlled parallel group trial”[8]. This study validates a novel treatment aimed at reducing collateral ventilation caused by an incomplete fissure. This is done by treating the pulmonary segment that covers the incomplete fissure with an endoscopic polymer foam. Selecting the right patient and the correct target segment for such a procedure is therefore a crucial part of treatment success which can only be accurately quantified using a CT scan.
Beyond the algorithm
As with all AI applications, developing a very robust algorithm requires much more than availability of data and ability to build one. It requires a thorough understanding of the patient population and the disease, understanding of what challenges you can encounter, and how the algorithm deals with it. Only then can one define markers that are robust across the board, having the ability to measure changes over time quickly and with high precision.
And yet, it’s not only about having a well performing algorithm. Defining the right set of measurements together with a deep understanding of the outcomes and the ability to interpret the results in the right context are critical factors determining the success of applying imaging in pulmonary trials.
Whether you are involved in developing new treatments for disease areas where CT is already an accepted modality to include in clinical trials, or yet being considered, it is equally valuable to understand how to do it effectively and maximize your return on investment.
If you are representing a CRO, Pharmaceutical or MedTech company and struggling with applying image analysis to your pulmonary trials effectively, or wondering where to start, feel free to reach out to us and get your questions answered.