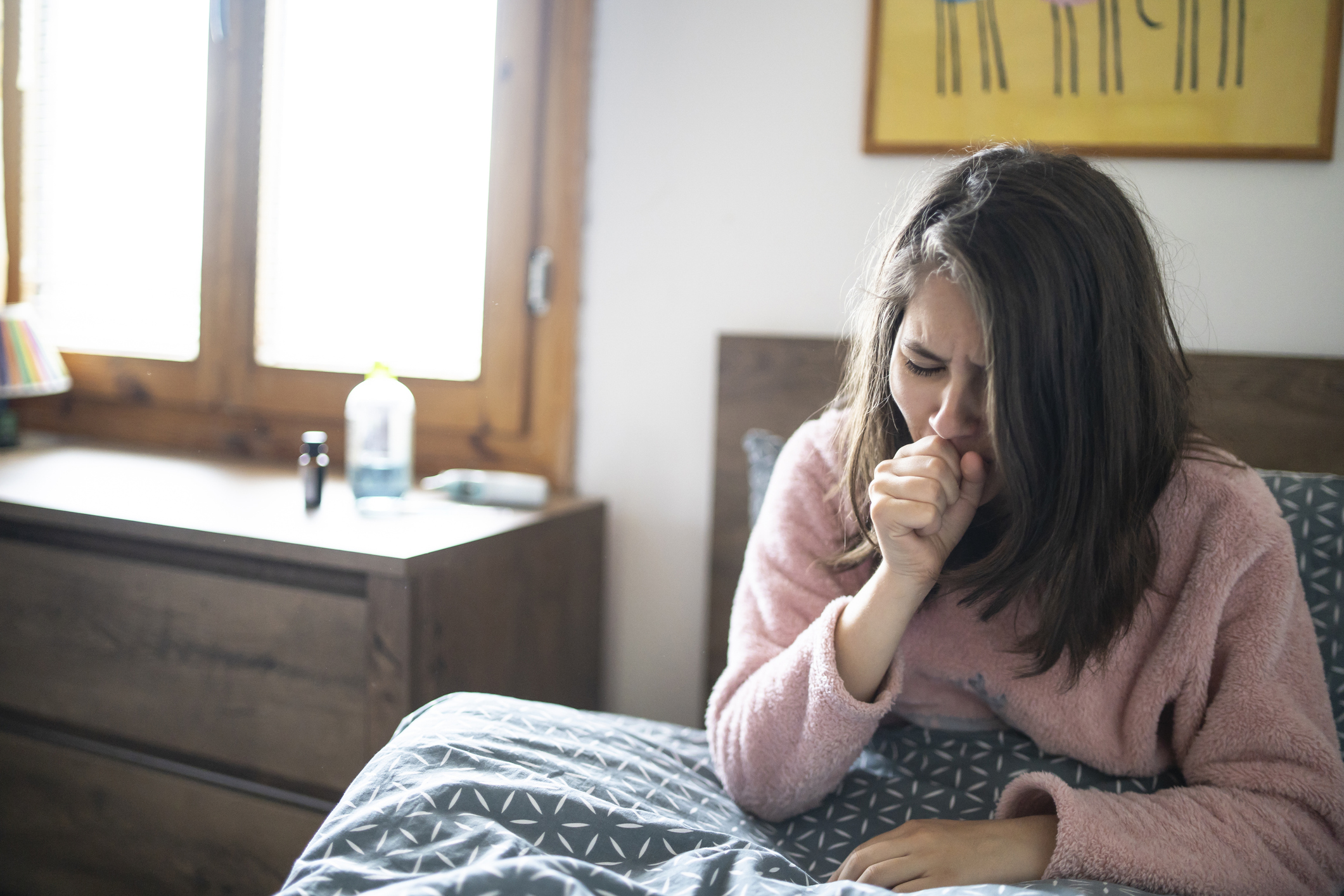
How can automatic detection of mucus plugs combined with a quantitative assessment of wall thickening provide a full picture of mucus impaction? Mucus impaction is a prevalent characteristic in many lung diseases. Understanding it is crucial to make fully informed treatment decisions. In this blog, we discuss mucus, challenges associated with it and how AI can facilitate the process of mucus treatment and intervention development.
Understanding mucus impaction is important in the treatment of various lung diseases
Mucus impaction plays an important role in many lung diseases. Serving as a protective barrier in healthy lungs, trapping dust, microbes and other foreign particles from entering the lungs, it can become thicker in response to infection, inflammation, or other lung conditions.
In the case when mucus production is increased, and/or the natural clearance through coughing is impaired, an excessive accumulation will occur, resulting in thickening of the airway walls and reduction in the airway lumen, eventually leading to a formation of obstructive mucus plugs.
Mucus impaction can manifest differently depending on the condition. For instance, in cystic fibrosis (CF) impaired mucociliary clearance results not only in mucus accumulation, but also the progressive damage of the airways. In Chronic Obstructive Pulmonary Disease (COPD) patients also suffer from impaired mucociliary clearance. They frequently experience periods of excess mucus production, and difficulties clearing the sputum from the lung, leading to occlusion of airways. In COPD, mucus plugging has even been associated with high mortality rates [1]. Also, in asthmatic patients, and specifically in severe asthma, there can be excessive mucus production leading to airway wall thickening and airflow obstruction. In bronchiectasis, mucus is contributing to bacterial infections, chronic inflammation and exacerbations, characterized by irreversible dilation and thickening of the airways.
To be fully informed in treatment decisions regarding mucus, it is important to understand the complications and changes in lung structure and function that may come with it.
Challenges in assessing and monitoring mucus impaction
The specific mechanisms by which the mucus contributes to disease progression are not fully understood. Being able to quantify mucus impaction on chest CTs would facilitate the development of more effective treatments, and targeted interventions.
Although computed tomography is a very sensitive and non-invasive method allowing for visualization of mucus impaction in both large and small airways, it is a major challenge to visually measure airway wall thickening, airway widening (bronchiectasis) and to detect mucus plugs based on chest CTs. The airway wall thickness as can be observed on a chest CT, represents thickening of the wall caused by inflammation but also of the mucus adhered to the wall.
It has been shown that it is very difficult for the human eye to accurately assess airway wall thickening and widening throughout the bronchial tree. Severe airway wall thickening in the more central large airways can be recognized relatively easily. However, the subtle changes in wall thickness of the numerous small airways are difficult to identify. To execute actual measurements of the airway wall thickness and width of all bronchi visible on a chest CT is nearly impossible as it is extremely time consuming. The same goes for the identification of mucus plugs.
Reliable quantification techniques to measure airway wall dimensions and to detect mucus plugs are essential for diagnosing airways disease as well as for monitoring of the treatment effects.
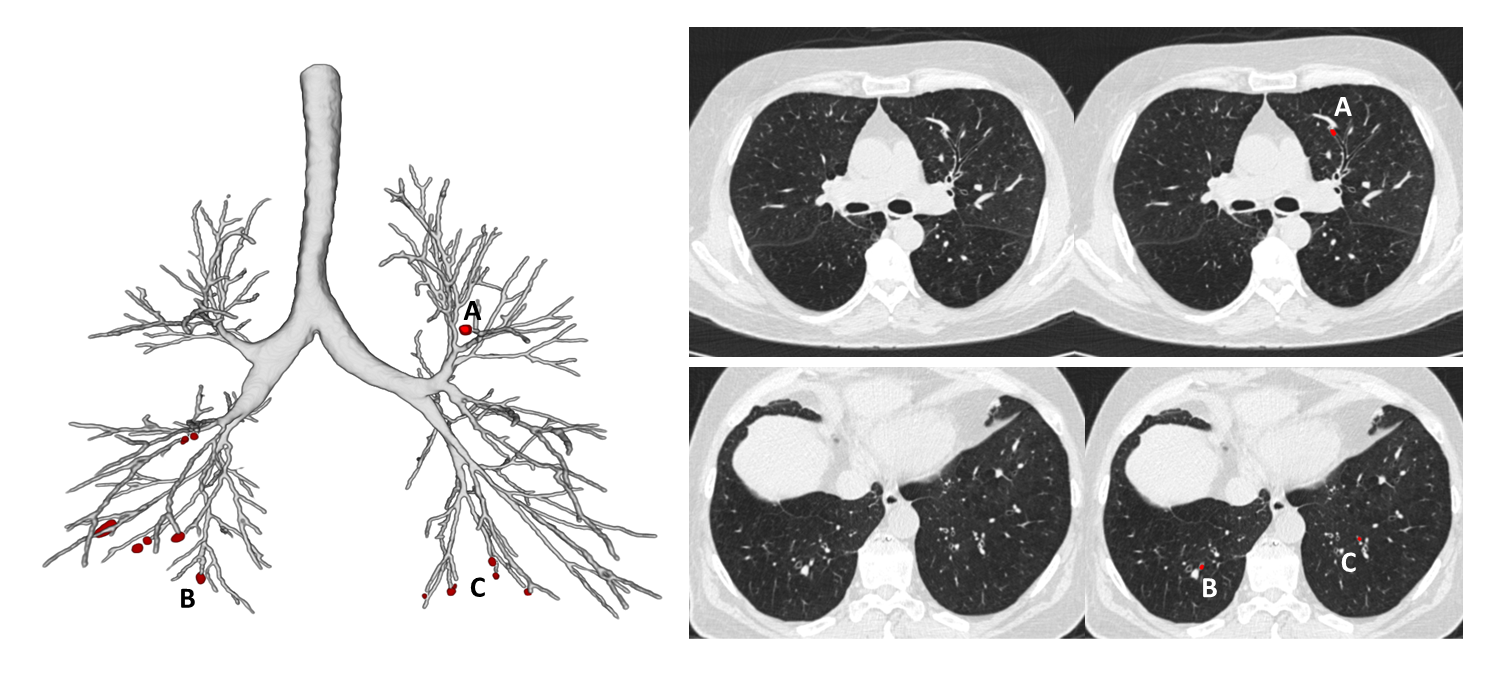
Figure 1
Mucus plug identification from chest CT
Comprehensive picture of mucus with AI
As mucus treatments can aim to either reduce mucus production, thinning it for easier clearance, or to address the underlying condition, a better understanding of the contribution of mucus impaction in patients can facilitate the development of novel targeted therapies to improve lung function and quality of life for patients with these diseases.
With the precision and robustness that deep learning technology brings to the table, we can recognize and precisely quantify details that could not be detected before. We are now able to look deep into the entire bronchial tree, and measure all relevant airway dimensions up to the smallest bronchi. This allows us to obtain objective, accurate, and sensitive information of all the bronchi visible in the scan in a matter of minutes. In addition, AI algorithms are much faster and more precise in detecting, localizing and counting mucus plugs (Figure 1).
The airway-artery (AA) ratio is the clinical standard for manually evaluating the condition of bronchi visible on a chest CT. Thirona's AI-based LungQ Bronchus-Artery (BA) algorithm (Figure 2), has been developed in line with this standard. It is able to provide precise AA dimensions, based on a large number of measurements across a multitude of points throughout the structures, allowing us to compute their exact ratios. These measurements deliver crucial information on disease severity and extent. The high sensitivity and reliability of BA algorithm measurements makes it an important tool for monitoring treatment effects over time, as it can detect small changes that are extremely difficult to identify with the human eye.
The BA algorithm was initially developed and validated in cystic fibrosis (CF), characterized by severe structural lung damage and thus very challenging to assess. Next, the BA-algorithm showed exceptionally high performance in other diseases with less severe structural changes such as COPD, severe asthma and bronchiectasis.
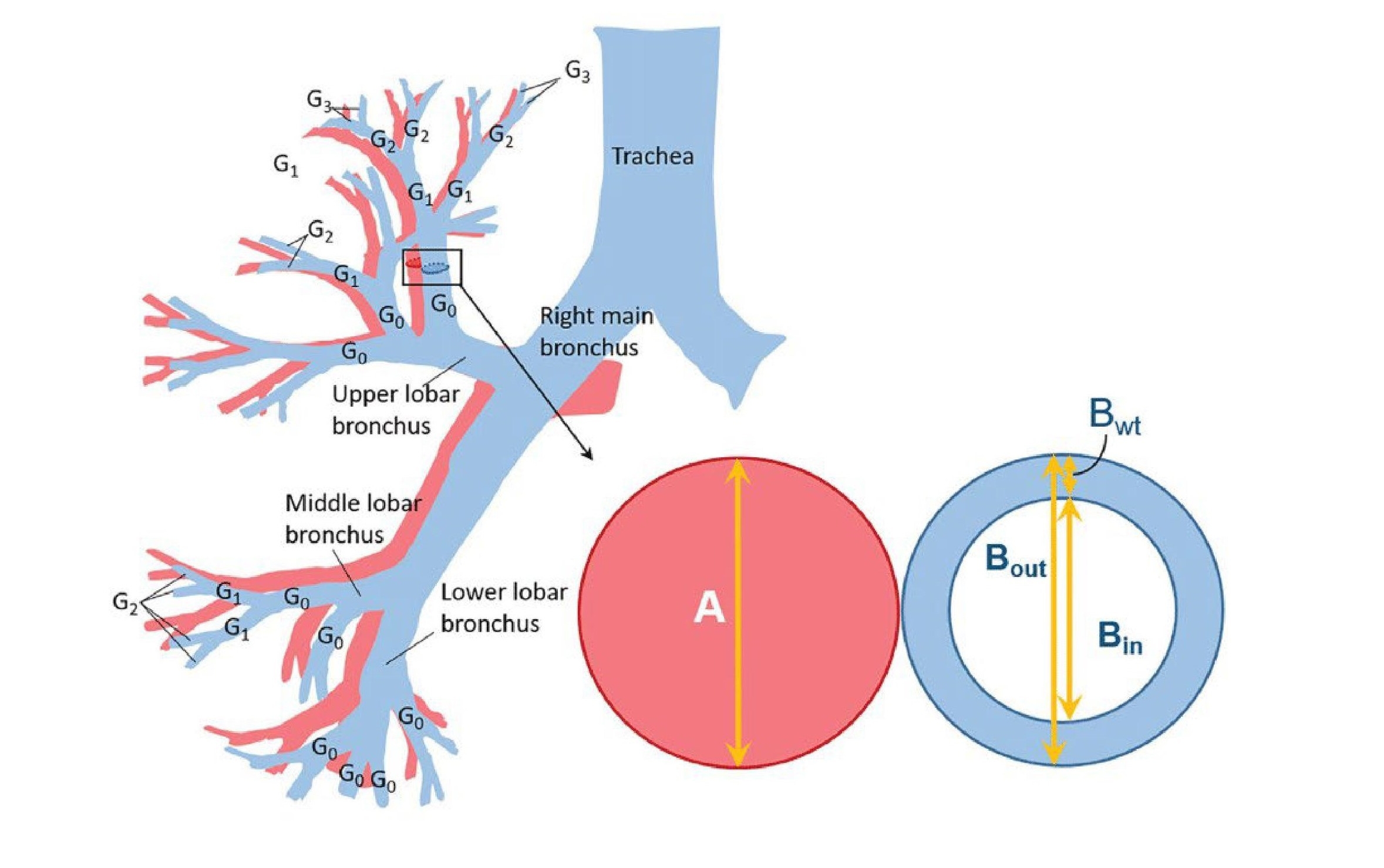
Figure 2
LungQ™ Bronchus-Artery Analysis
In addition to the advanced BA-analysis, Thirona developed an algorithm that can conduct a fully automatic mucus plug quantification across the airway tree, up to the peripheral branches. In combination with BA analysis, it offers the most sensitive way to provide a complete picture on occlusive and non-occlusive mucus accumulation
By making use of state-of-the-art segmentation algorithms, it is possible to extract the above described key metrics on a lobar and sub-lobar level, as well as per airway generation, altogether enabling a full assessment of mucus plugs, bronchial wall thickening, bronchial narrowing andother abnormalities that come with mucus impaction
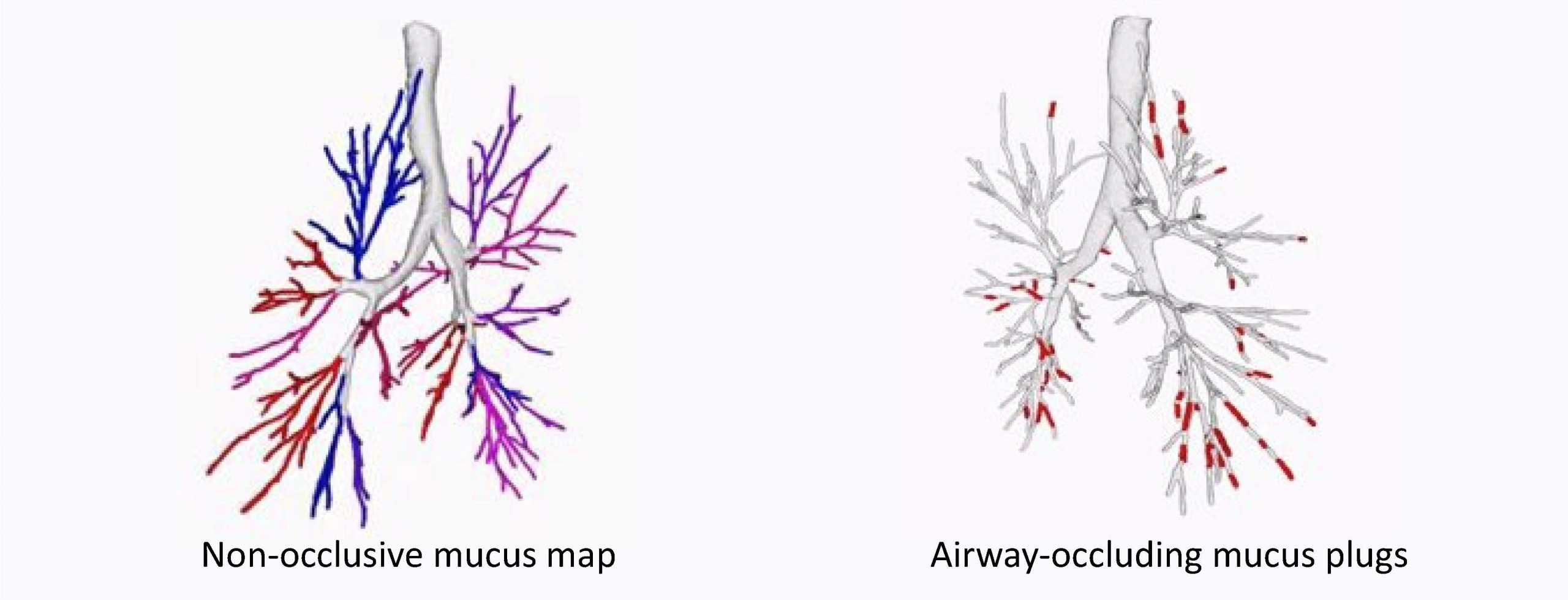
Figure 3
Comprehensive assessment of mucus development with LungQ™ BA & Mucus
Innovation potential for personalized treatment
The comprehensive understanding of mucus dynamics can significantly contribute to the development of more effective treatments for lung diseases like COPD, CF, asthma and bronchiectasis.
Greater precision and sensitivity in quantification and localization of mucus plugs even in peripheral branches, forms a strong basis for targeted therapies and personalized treatment approaches tailored to individual patients’ mechanisms of mucus impaction. Additional insights into factors contributing to mucus impaction can also inform the design of drug delivery systems, enhancing effective penetration and disperse of mucus in the airways.
Having the ability to provide objective quantitative evidence on disease progression and drug efficacy, AI will enhance treatment monitoring and assessment techniques in the long run.
In sum, by addressing mucus obstruction more effectively, AI-enabled treatment strategies can help reduce the frequency and severity of exacerbations in patients with mucus obstructive lung diseases, ultimately leading to better long-term disease management and improved quality of life.
Feel free to contact Thirona’s expert team to learn more on our capabilities in bronchi and mucus analysis.
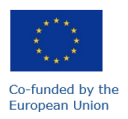
Co-funded by the European Union. Views and opinions expressed are however those of the author(s) only and do not necessarily reflect those of the European Union or European Innovation Council and SMEs Executive Agency. Neither the European Union nor the granting authority can be held responsible for them.